Integrating Cloud AI & ML: A Guide for Startup Decision Makers
A comprehensive exploration of how startups can harness cloud-based AI and ML services effectively, covering the nuances of selecting the right services, aligning them with business goals, addressing financial and technical challenges, and ensuring ethical and future-focused deployment.
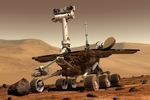
The technological landscape is shifting rapidly, and at the epicentre of this revolution is the amalgamation of cloud computing with artificial intelligence (AI) and machine learning (ML). Startups, known for their agility and innovation, stand at a vantage point to harness this combined power. But how can startup decision-makers make the best of these technologies?
Deciphering Cloud-based AI & ML Services
Navigating the dynamic world of AI and ML, especially with cloud services, requires a deep dive into the myriad offerings and terminologies. Startups need a clear understanding to tailor these tools to their unique business needs.
At its core, AI seeks to emulate human thought processes in a computerized model. This involves creating algorithms and models that empower computers to undertake tasks that, until now, solely reside in the realm of human cognition. These tasks range from recognizing intricate patterns in vast data sets to making predictive decisions based on evolving data. Meanwhile, ML, a crucial subset of AI, is the science of designing and applying algorithms that let computers learn from and make data-based decisions. ML's ability to change its behaviour based on the data it processes without human intervention makes it distinct.
Today's cloud ecosystem has several significant players offering many AI and ML services tailored to varied needs. Companies like Amazon Web Services (AWS) offer tools like SageMaker for accessible ML model building and training. Microsoft's Azure boasts a suite of AI services that can integrate with apps, websites, and bots to simulate human intelligence. On the other hand, Google Cloud's AI and ML tools are known for their scalability and deep learning capabilities. These platforms provide a holistic environment that covers the entire AI and ML project lifecycle – from data preprocessing and model training to deployment and monitoring.
But it's not just about the big names; numerous other cloud providers offer niche solutions, plugins, and integrations that cater to specific industry needs. Moreover, the open-source community actively contributes tools and platforms that can be integrated into cloud environments, ensuring startups have vast resources. Startups must discern which tools align best with their objectives, whether they need pre-trained models or require tools to train custom models from scratch, and what scalability and flexibility they expect as their data needs grow.
Furthermore, understanding the economic implications of these services is crucial. Different cloud providers have varying pricing models, from pay-as-you-use to subscription models. Factoring in costs, especially for startups operating on tight budgets, ensures they extract maximum value from their AI and ML investments.
In essence, deciphering the cloud-based AI and ML landscape is akin to piecing together a jigsaw puzzle, where each piece, whether a tool, platform, or service, must fit perfectly to reveal the bigger picture - a fully optimized, AI-driven business model.
Strategic Integration in Business Verticals
Harnessing the potential of AI and ML across diverse business verticals can have transformative implications, unlocking efficiencies and insights that were previously inaccessible.
Sales and Marketing: AI and ML integration can be a game-changer in the sales and marketing space. Beyond traditional analytics, AI-driven solutions can offer deep insights into consumer behaviour, surfacing patterns that might be indiscernible to human analysts. ML models help businesses tailor their marketing strategies for maximum impact by predicting future purchase behaviours and identifying emerging market trends. Moreover, AI can assist in real-time pricing optimization, adjusting prices based on instantaneous market dynamics or even a specific customer's purchasing history. Dynamic content generation, powered by AI, can also craft personalized marketing messages on-the-fly, ensuring that each user engagement feels individualized and relevant.
Operations: Operational efficiency stands at the core of a successful startup, and AI and ML can push this to new heights. AI can optimize supply chain dynamics, predict disruptions, and suggest real-time alternatives. Inventory management, traditionally labour-intensive, can be streamlined using ML models that predict stock needs based on historical data, seasonality, and market trends. Furthermore, logistics – a complex dance of variables – can be made more efficient. ML algorithms can predict optimal delivery routes, considering traffic patterns, weather conditions, and package prioritization, ensuring timely deliveries with reduced overheads.
Human Resources: The human aspect of businesses, while crucial, is fraught with challenges and inefficiencies. However, AI and ML stand poised to revolutionize HR processes. Recruitment, for instance, can be supercharged with AI tools that screen through thousands of resumes in minutes, highlighting the most relevant candidates based on predefined criteria. Beyond just skill matching, ML models can analyze historical data to predict a candidate's potential cultural fit and their likely tenure at the company, aiding in retention strategies. Employee engagement and training modules can also be personalized using AI, tailoring content to suit individual employees' learning styles and pace.
Product Development: AI can be an invaluable ally in product development. Startups can use ML to analyze customer feedback, identify common pain points or feature requests, and inform the product roadmap. AI-driven prototyping tools can simulate user interactions with new features, offering insights before full-fledged development begins. Additionally, predictive analytics can help forecast the market reception of new product launches, aiding inventory and marketing decisions.
Customer Support: In today's digital age, customer support has evolved beyond call centers. AI-powered chatbots and virtual assistants take the frontline in handling customer queries, providing instant responses, and ensuring 24/7 support. These bots can be trained using ML to manage more complex questions over time, continually improving their efficiency. Moreover, sentiment analysis tools can gauge customer satisfaction in real-time during interactions, allowing for instantaneous course corrections and ensuring a superior customer experience.
Financial Implications for Startups
The move to incorporate cloud-based AI and ML solutions is as much a financial endeavour as a strategic one. With the promise of game-changing results, it's essential to understand the investment landscape thoroughly to derive the maximum ROI and ensure sustainable growth.
Traditional AI and ML setups, especially those built from scratch, often involve significant upfront capital expenditure. This includes costs related to acquiring robust hardware, licenses for proprietary software, and potential consultancy fees for specialized expertise. For startups, this could mean a substantial chunk of their capital being earmarked for just the initial setup, potentially straining their financial resources.
However, the advent of cloud-based AI and ML services has fundamentally altered this financial calculus. Instead of hefty upfront costs, cloud providers typically operate on a pay-as-you-go or subscription-based model. This shift in cost structure is particularly advantageous for startups. It allows them to avoid large initial outlays and provides the flexibility to scale their spending based on actual usage and needs. This flexibility is invaluable, as startups often face fluctuating demands, and being tied down to a fixed cost can be detrimental.
Beyond the direct costs associated with AI and ML tools, startups should also factor in the potential operational savings these technologies can usher in. For instance, automating manual processes with AI can significantly reduce labour costs. ML-driven predictive analytics can optimize inventory levels, leading to savings on storage costs and reducing wastage. Furthermore, improved decision-making, informed by AI insights, can lead to better allocation of resources, maximizing revenue opportunities and minimizing unproductive expenditures.
Then there's the aspect of opportunity costs. In the fast-paced startup ecosystem, time is often as valuable as money. Due to a lack of insights or inefficiencies, delays in product launches or market entries can have significant financial implications. By harnessing the power of AI and ML, startups can accelerate their go-to-market strategies, capitalize on emerging trends, and stay ahead of competitors, ensuring they don't leave money on the table.
Finally, while considering the financial landscape, it's imperative to account for the potential hidden costs. These could arise from data migration, training the team to use new tools or even the need for periodic recalibration and fine-tuning of AI models. Having a holistic view of the financial journey, factoring in all potential costs and savings, will empower startups to make informed decisions and strategically allocate their precious capital.
Technical Integration and Challenges
Leveraging cloud-based AI and ML services presents many opportunities for startups, but the road to seamless technical integration is often challenging. Addressing these hurdles head-on, with a well-structured approach, is crucial for ensuring a successful AI transformation.
One of the primary considerations is Data Compatibility. Startups often have data accumulated from various sources, in different formats, and of varying quality. Before AI and ML algorithms can work their magic, this data must be cleaned, normalized, and possibly reformatted. In some cases, legacy data might be in a format that's not directly compatible with modern cloud-based AI tools, necessitating conversion tools or manual intervention. This can be time-consuming and may introduce potential points of failure or data loss.
Another crucial aspect is the Scalability of the solutions. Startups, especially those in a rapid growth phase, can experience exponential increases in data volume. The AI and ML solutions must handle this growth without significant reconfiguration or downtime. This necessitates choosing platforms and services that inherently support scalability in data processing and storage.
Security Concerns also come to the fore when integrating AI and ML into business operations. Data transfer to and from the cloud, especially if it involves sensitive customer or business information, needs to be secure. Employing end-to-end encryption, secure access protocols, and robust firewall protections are vital. Additionally, ensuring compliance with data protection regulations, like GDPR or CCPA, is essential to avoid potential legal ramifications and maintain customer trust.
Then there's the challenge of Model Validity. The landscape of AI and ML is continually evolving, with new algorithms and models emerging regularly. The models chosen today might become obsolete or less efficient over time. Startups need to ensure a system of periodic review, validation, and potential updating of their AI models to maintain accuracy and efficiency. This dynamic nature of AI demands continuous learning and agility.
Integration with Existing Systems poses another challenge. Startups might already have various IT systems, CRM tools, ERP solutions, or custom-built software. The AI and ML solutions must seamlessly integrate with these without disrupting existing workflows. This might require custom APIs, middleware solutions, or even some restructuring of current IT architectures.
Lastly, while cloud-based AI and ML services offer the advantage of off-premises processing power, there's the challenge of Latency. Real-time applications, like AI-driven chatbots or instant data analytics, require swift data transfer rates. Startups must ensure they're geographically close to their cloud provider's data centers or employ edge computing solutions to minimize latency.
Ethical Considerations and Responsible AI
In the age of ubiquitous AI and ML applications, the ethical considerations surrounding these technologies have never been more pressing. As we inch closer to realizing the vast potential of AI, it becomes imperative for startups to grapple with the complex ethical landscape accompanying such progress. Integrating AI responsibly into business operations isn't just about compliance or avoiding controversy; it's about fostering trust, ensuring fairness, and contributing positively to society.
- Bias and Fairness: At the core of many AI models is the data they're trained on. If this data carries biases, the AI system will inevitably perpetuate and potentially amplify these biases. For startups, this could translate to unfair product recommendations, skewed hiring practices, or misrepresentation in content curation. The challenge lies in recognizing these biases, actively seeking diverse datasets, and applying fairness corrections to ensure that AI systems operate equitably.
- Transparency and Explainability: A common criticism of many AI systems is their "black box" nature. Understanding how a particular AI model arrives at a decision can be crucial for users and stakeholders. Startups must invest in explainable AI, ensuring that their algorithms' decision-making processes are transparent and can be understood by those affected. This transparency bolsters trust and aids in debugging and refining the system.
- Data Privacy and Security: With AI systems often requiring vast amounts of data, concerns about user privacy are paramount. Startups must ensure robust data protection protocols, anonymize personal information where possible, and be transparent about data collection and usage practices. Moreover, ensuring compliance with global data protection regulations, such as GDPR, is non-negotiable.
- Accountability: As AI systems become more autonomous, determining accountability in cases of errors or mishaps can be challenging. Is it the developers, the users, the data providers, or the AI itself that's at fault? Startups must pre-emptively define responsibility matrices and ensure a clear line of accountability for AI-driven actions. This clarity is essential not just for legal reasons but also for refining and improving systems.
- Human-AI Collaboration: The future of AI doesn't lie in replacing humans but in augmenting human capabilities. Ethical considerations must also ensure that AI systems are designed to collaborate with humans, providing tools that enhance decision-making, creativity, and productivity rather than overshadowing or sidelining human roles.
- Societal Impact: Beyond immediate business operations, startups must also reflect on the broader societal impact of their AI applications. This includes considering potential job displacements, the implications of deepfake technologies, or the societal divides that unchecked AI could exacerbate. A holistic view that factors in societal well-being can guide startups toward more responsible and inclusive AI deployments.
Looking Ahead: The Future of Cloud AI & ML for Startups
As we peer into the horizon, it's evident that cloud-based AI and ML services are not just a fleeting trend but are setting the stage for the future of business operations, especially for agile startups. The intersection of cloud technologies with AI and ML is forging a new frontier, reshaping industries and redefining what's possible for entrepreneurial ventures.
Democratization of Advanced Technology: One of the most profound impacts of cloud-based AI and ML services is democratizing cutting-edge technologies. Previously, only large corporations with vast resources could access and leverage advanced AI capabilities. However, cloud platforms level the playing field, enabling even the smallest startups to tap into powerful AI and ML algorithms. This democratization means that innovation is no longer confined to tech giants; startups from any sector can be at the forefront of AI-driven breakthroughs.
Hyper-Personalization: As data becomes the new oil, its refining process – through AI and ML – will lead to hyper-personalization in products and services. Startups can offer unique experiences to individual users based on their preferences, behaviours, and past interactions. Whether it's e-commerce platforms suggesting products, content streaming services curating playlists, or fintech solutions offering personalized financial advice, the age of one-size-fits-all is giving way to bespoke experiences.
AI-driven Decision Making: The future will significantly shift towards AI-driven decision-making processes. With the exponential growth of data, human-driven analysis will become increasingly impractical. Startups will rely on AI to sift through vast datasets, draw insights, and even make predictive forecasts. This will speed up decision-making and introduce a higher degree of accuracy, reducing risks and enhancing the probability of success in various ventures.
Evolution of Business Models: As AI and ML continue to permeate various functions, we can anticipate a metamorphosis in traditional business models. Companies might evolve from product-centric approaches to service-centric ones facilitated by AI. For example, a startup that once sold fitness wearables might transition to offering holistic health management platforms, using AI to analyze data from wearables and provide personalized health advice.
Ethical and Regulatory Landscape: Great power comes with great responsibility. The pervasive integration of AI will inevitably lead to discussions about ethics, privacy, and regulations. There will be increased scrutiny on how AI models make decisions, the biases they might inherit, and the privacy implications of widespread data collection. Startups must be at the forefront of championing ethical AI, ensuring transparency in their algorithms, and actively participating in shaping a fair regulatory landscape that fosters innovation.
Collaborative AI Ecosystems: The future will likely witness a rise in collaborative ecosystems where startups, despite being in competitive domains, share specific AI resources, tools, or datasets to drive mutual growth. This collaboration can spur faster innovation, reduce redundancies, and lead to the emergence of holistic solutions that cater to broader market needs.
In Conclusion
In an era of rapid technological advancement, startups have a golden opportunity. They can drive growth, innovation, and efficiency by strategically integrating cloud-based AI and ML into their operations. It's a journey filled with challenges, but with the right approach, the rewards are substantial.
Your Pilot in the Cloud
Looking for expert help designing your cloud architecture or planning and executing your migration projects? Contact us today!
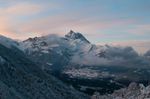
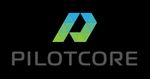
Your Pilot in the Cloud
Contact us today to discuss your cloud strategy! There is no obligation.
Let's Talk